Ensemble generation
For predictions on all timescales, initial perturbations among an ensemble (representing our estimates of uncertainty in observing different components of the Earth System) grow with lead time and provide an estimate of uncertainty in forecast outcomes. For reliable forecasts, the forecast uncertainty needs to be representative of observed outcomes. In practice, however, it is generally agreed that ensemble prediction systems (EPSs) on S2S timescale do not have enough spread among ensemble members, and consequently, are overconfident (see example in Figure 4). Therefore, efforts need to be made to improve the representation of uncertainty in initial conditions (using methods such as singular vector, bred vector, lagged averaging) or to develop methods (such as stochastic parameterization) to enhance spread during model integration.
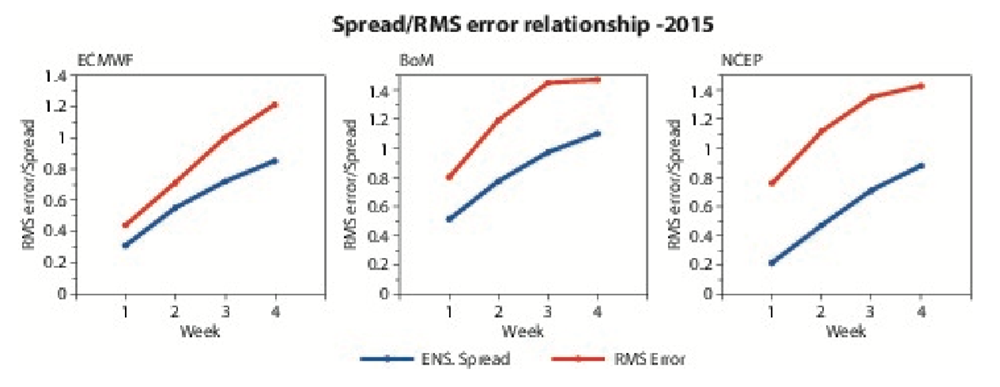
Figure 4. Evolution of MJO ensemble spread (blue lines) and Root mean square Error (RMSE) (red lines) as a function of lead time (in weeks) for the all the ECMWF (left panel), BoM (middle panel) and NCEP (right panel) real-time ensemble forecasts produced in2015. The RMSE has been calculated using ERA Interim.
Although operational sub-seasonal EPSs are based on our current state of knowledge, the influence of initialization and ensemble configuration on a sub-seasonal timescale is neither well understood nor well researched. As one of the foci for the next phase of the S2S project, research on the ensemble generation techniques need to engage in addressing following challenges:
- Optimal initial-perturbation strategies for the sub-seasonal timescale have not been systematically studied.
- In the sub-seasonal timescale, the many models suffer from over-confident predictions. The reason for the discrepancy between the observed and forecast spread may be result from both random and systematic errors, in contrast to short-range predictions in which the random error is dominant; the relative importance of the different causes is not well understood.
- For certain regimes (e.g. MJO, tropical cyclone), there might be skill improvement from initial perturbations of the ocean in addition to the atmospheric perturbations, however, the coupled initialization techniques are in their infancy and have not been fully researched.
- While stochastic parameterizations schemes have the potential to improve forecast performance, little research on their impact on this timescale has been conducted. With this in mind, and with the availability of the S2S database and the possibility of additional coordinated forecast experiments, we propose tackle the above-mentioned challenges. The research agenda will be developed in close collaboration with the PDEF Working Group and WGNE.
Proposed studies
- Study the influence of forecast configuration strategies, including initialization strategies used in the current generation of S2S prediction systems (burst and lagged ensemble) on the forecast spread.
- Benchmark the spread-error relationship in the current generation of S2S prediction systems.
- Explore the impacts of coupled initial perturbations on the sub-seasonal prediction, and develop techniques of coupled initial perturbations.
- Investigate the impact of stochastic parameterizations for the sub-seasonal prediction.